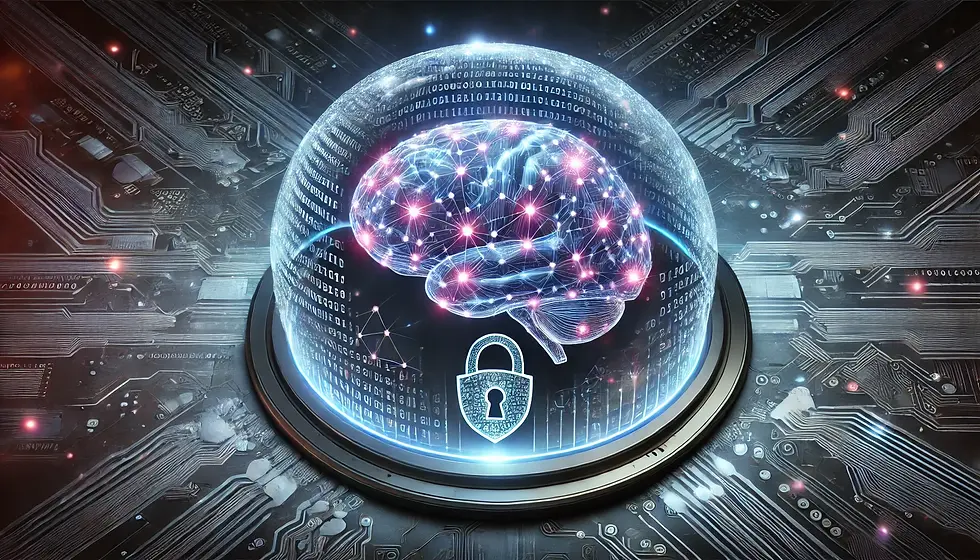
In an era where cyber threats evolve at breakneck speed, the future of cybersecurity hinges on our ability to stay one step ahead. Automated Security Validation (ASV) has emerged as a cornerstone of modern cybersecurity strategies, enabling organizations to continuously assess and improve their security posture. As we look to the horizon, the integration of Artificial Intelligence (AI) and Machine Learning (ML) into ASV promises to revolutionize how we approach cybersecurity. This blog post explores the cutting-edge developments in ASV and how they're shaping the future of digital defense.
The Current State of Automated Security Validation
Before we dive into the future, let's briefly recap where we stand today with ASV:
Key Features of Current ASV Solutions:
Continuous Testing: ASV tools like Pentera provide ongoing assessment of an organization's security measures, rather than point-in-time snapshots.
Real-World Attack Simulation: These tools mimic actual hacker techniques to identify vulnerabilities that might be exploited in real attacks.
Comprehensive Coverage: ASV can test across a wide range of systems, applications, and network configurations.
Automated Reporting: Detailed reports are generated automatically, highlighting vulnerabilities and suggesting remediation steps.
Integration Capabilities: Many ASV tools can integrate with existing security infrastructure for streamlined operations.
While these features have significantly enhanced cybersecurity efforts, the rapid evolution of cyber threats demands even more sophisticated solutions.
The AI and ML Revolution in Automated Security Validation
The integration of AI and ML into ASV is not just an incremental improvement—it's a paradigm shift. Here's how these technologies are set to transform ASV:
1. Enhanced Threat Intelligence
Current Limitation: Traditional ASV tools rely on predefined attack scenarios and known vulnerabilities.
AI/ML Solution: Machine learning algorithms can analyze vast amounts of global threat data in real-time, identifying new attack patterns and emerging threats before they become widespread.
Impact: This proactive approach allows organizations to prepare for and mitigate potential threats before they're exploited in the wild.
2. Adaptive Attack Simulations
Current Limitation: Many ASV tools use a relatively static set of attack simulations.
AI/ML Solution: AI-driven ASV can dynamically adapt attack simulations based on the specific environment, learning from each test to create more sophisticated and relevant attack scenarios.
Impact: This results in more realistic and effective testing, uncovering vulnerabilities that might be missed by more rigid testing methods.
3. Predictive Vulnerability Analysis
Current Limitation: Traditional tools focus on identifying existing vulnerabilities.
AI/ML Solution: Machine learning models can analyze system configurations, code patterns, and other factors to predict potential future vulnerabilities.
Impact: Organizations can address security weaknesses before they become exploitable, significantly reducing their attack surface.
4. Automated Remediation Prioritization
Current Limitation: Security teams often struggle to prioritize the multitude of vulnerabilities identified by ASV tools.
AI/ML Solution: AI algorithms can assess the criticality of each vulnerability based on factors like exploitability, potential impact, and relevance to the specific organization.
Impact: This enables security teams to focus their efforts on the most critical issues first, maximizing the efficiency of their remediation efforts.
5. Behavioral Anomaly Detection
Current Limitation: Current ASV tools may miss subtle, behavior-based vulnerabilities.
AI/ML Solution: Machine learning can establish baselines for normal system and user behavior, flagging anomalies that might indicate a security risk.
Impact: This approach can uncover insider threats, compromised accounts, and other security issues that traditional vulnerability scans might miss.
6. Natural Language Processing for Policy Compliance
Current Limitation: Assessing compliance with security policies often requires manual review.
AI/ML Solution: Natural Language Processing (NLP) can analyze security policies and configurations, automatically flagging potential compliance issues.
Impact: This streamlines the compliance process, reducing the risk of human error and ensuring more consistent policy enforcement.
7. Quantum-Resistant Cryptography Testing
Current Limitation: Most ASV tools aren't prepared for the potential threat posed by quantum computing.
AI/ML Solution: Advanced AI models can simulate quantum computing attacks, testing the resilience of current cryptographic methods.
Impact: Organizations can proactively strengthen their encryption methods to withstand future quantum-based attacks.
Real-World Applications and Case Studies
To illustrate the potential of AI and ML in ASV, let's explore some hypothetical case studies based on emerging trends:
Case Study 1: Financial Institution Thwarts Zero-Day Attack
A large bank implemented an AI-enhanced ASV system that continuously analyzed global threat intelligence. The system identified a new attack pattern targeting a specific type of financial software. Before any public disclosure of the vulnerability, the AI suggested a tailored attack simulation. This proactive approach allowed the bank to identify and patch the vulnerability, effectively preventing a potential zero-day attack.
Key Takeaway: AI-driven threat intelligence in ASV can provide a critical time advantage in cybersecurity.
Case Study 2: Healthcare Provider Enhances HIPAA Compliance
A healthcare organization struggled with maintaining HIPAA compliance across its complex IT infrastructure. They deployed an ASV solution with NLP capabilities to analyze their security policies and system configurations. The AI-powered system automatically identified several subtle compliance issues that had been overlooked in manual audits, allowing the organization to rectify these issues promptly.
Key Takeaway: AI can significantly enhance the accuracy and efficiency of compliance-related security validations.
Case Study 3: Tech Startup Predicts and Prevents Future Vulnerabilities
A rapidly growing tech startup used an ML-based ASV tool that analyzed their development practices and code patterns. The system predicted several potential vulnerabilities in their upcoming software release. By addressing these issues in the development phase, the company avoided a costly post-release patching process and potential security breaches.
Key Takeaway: Predictive vulnerability analysis can shift cybersecurity from a reactive to a proactive stance.
Challenges and Considerations
While the future of AI and ML in ASV is promising, it's not without challenges:
Data Privacy Concerns: The effectiveness of AI/ML models often depends on large datasets, which can raise privacy issues, especially in heavily regulated industries.
False Positives: As AI systems become more sensitive to potential threats, there's a risk of increased false positives, which could overwhelm security teams.
AI-Powered Attacks: As defenders use AI to enhance security, attackers are also leveraging AI to create more sophisticated threats.
Skill Gap: The cybersecurity industry already faces a significant skills shortage, and the integration of AI/ML may exacerbate this issue.
Ethical Considerations: The use of AI in simulating attacks raises ethical questions, particularly when it comes to the potential for these tools to be misused.
Explainability: Some AI models, particularly deep learning systems, can be "black boxes," making it difficult to explain their decision-making process. This can be problematic in security contexts where transparency is crucial.
Preparing for the Future of ASV
As we look towards this AI-driven future of ASV, organizations can take several steps to prepare:
Invest in Data Infrastructure: The effectiveness of AI/ML in ASV relies heavily on data. Organizations should focus on collecting, storing, and managing high-quality security data.
Develop AI/ML Expertise: Start building or acquiring talent with expertise in AI and ML, particularly as it applies to cybersecurity.
Implement Ethical Frameworks: Develop clear ethical guidelines for the use of AI in security testing to ensure responsible use of these powerful tools.
Enhance Collaboration: Foster closer collaboration between security teams, data scientists, and AI specialists to drive innovation in ASV.
Stay Informed: The field of AI in cybersecurity is rapidly evolving. Regularly engage with the latest research and attend relevant conferences to stay updated.
Start Small, Scale Up: Begin with pilot projects to implement AI/ML in specific areas of your security validation process, then scale based on lessons learned.
Focus on Interpretability: When selecting or developing AI-enhanced ASV tools, prioritize solutions that offer explainable AI, allowing for better understanding and trust in the system's decisions.
The Role of Human Expertise
While AI and ML are set to revolutionize ASV, it's crucial to remember that these technologies are tools to enhance, not replace, human expertise. The future of ASV will likely be a symbiotic relationship between AI systems and human security professionals:
AI/ML Systems: Will handle data analysis, pattern recognition, and routine testing at a scale and speed impossible for humans.
Human Experts: Will provide context, make nuanced decisions, and drive the strategic direction of security efforts.
This collaboration will allow organizations to leverage the strengths of both artificial and human intelligence, creating a more robust and adaptive security posture.
Looking Ahead: Emerging Trends in ASV
As we peer into the future, several exciting trends are emerging that could further transform ASV:
Quantum Computing in ASV: While quantum computing poses a threat to current encryption methods, it also offers potential for incredibly fast and complex security simulations.
5G and IoT Security Validation: The proliferation of 5G and IoT devices will require new approaches to ASV that can handle the scale and diversity of these networks.
Cloud-Native ASV: As more organizations move to the cloud, ASV tools will evolve to provide deeper, more integrated testing of cloud environments.
Emotional AI in Social Engineering Tests: Advanced AI might simulate human emotions and behaviors more accurately, allowing for more realistic social engineering tests.
Digital Twin Technology: Creating digital twins of entire networks could allow for more comprehensive and risk-free security testing.
Conclusion: Embracing the AI-Driven Future of ASV
The integration of AI and ML into Automated Security Validation represents a significant leap forward in our ability to defend against cyber threats. By enhancing threat intelligence, enabling adaptive testing, predicting vulnerabilities, and streamlining remediation efforts, these technologies are setting the stage for a more proactive and resilient cybersecurity landscape.
However, this future is not without its challenges. Privacy concerns, the potential for misuse, and the need for transparency in AI decision-making are all issues that must be carefully navigated. Moreover, the human element remains crucial – AI and ML are powerful tools, but they require skilled professionals to wield them effectively.
As we stand on the brink of this new era in cybersecurity, organizations must prepare by investing in the right technologies, developing the necessary skills, and fostering a culture of continuous learning and adaptation. Those who embrace these changes will be best positioned to defend against the evolving threat landscape and ensure the security of their digital assets in the years to come.
At Silo City IT, we're committed to staying at the forefront of these developments, continuously evolving our ASV solutions to incorporate the latest AI and ML technologies. Our goal is to provide our clients with the most advanced, effective, and ethical security validation tools available, helping them navigate the complex and ever-changing world of cybersecurity with confidence.
The future of Automated Security Validation is bright, powered by the incredible potential of AI and ML.
As we move forward, let's embrace these technologies responsibly, always striving to create a safer digital world for all.
Comments